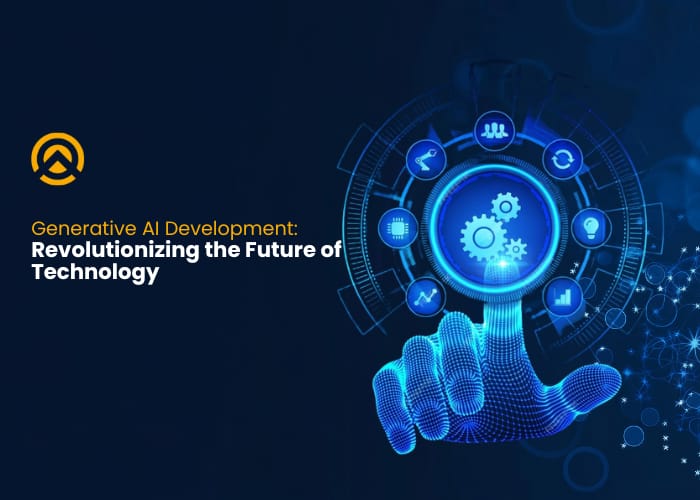
The expansion of AI is presenting various advancements in different fields and taking care that companies are required to integrate AI into their processes. In other words, organizations can cherish many advantages with the involvement of AI-integrated solutions. So, this is all about AI right when it comes to more advancements Generative AI development techniques being the sub-term of AI provide solutions that are more valuable than the traditional AI development approach. The further section explores more about generative AI and its expansion.
What is Generative AI?
Generative AI is defined as a set of algorithms that are used for the creation of new content in different formats. This can be present in the form of audio, code, images, text, videos, and simulations. These algorithms are majorly based on advanced concepts of AI like Generative Adversarial Networks (GAN), Transformer Models, and variational autoencoders (VAEs). The basic understanding of this technique is to understand the patterns and structures present in the datasets. These learning patterns result in effective content creation of different formats.
No doubt artificial intelligence is rapidly covering all the industrial sectors of the economy and to highlight its generative AI expansion, this article is constructed. In this we are covering major technical aspects of generative AI development, its industrial expansion in terms of applications, and its futuristic aspects in terms of technical advancements. So read this out for betterment in the generative AI development techniques.
The Innovative Techniques of Generative AI
The changes we see within the software industry are all due to the continuous integration of innovative techniques. The same goes with the field of AI and sub-technologies, so this section is all about highlighting these techniques that are working under the carpet.
1. Generative Adversarial Networks (GANs)
This is one of the key generative AI techniques that works by generating highly realistic content or data with the help of its two major neural networks. These are the generator and discriminator and both of them are trained together to generate effective responses. In this, the generator works on creating new data while the discriminator works on evaluating generated data in comparison to the real data sets. Along with the evaluation it provides feedback for the generator’s improvements.
2. Transformer Models
These transformer models are the backbones of generative AI development procedures and were first developed for Natural Language Processing (NLP). As such for a well-known model of GPTs (Generative Pre-Trained Transformer) for the creation of human-like-texts. These models adopt the self-attention approach for the processing of input sequences in parallel terms for the generation of relevant and coherent text generations.
3. Variational Autoencoders (VAEs)
These are the other types of generative AI models that work on learning to encode the input data into a latent space and then decode it back into the data format. This approach works on generating new data points with the help of sampling data from the latent space and makes that data useful in the required space.
Types of Applications Expected with Generative AI in Different Industries
The expansion of Generative AI techniques is everywhere and this not only lets the field grow exponentially but also enhances their technical expertise. Some of its technically advanced application areas are mentioned below.
1. Cybersecurity
The more advanced the technical terms are getting advanced the more we can see the expansion of cyber-attacks and breaches within the market. We can expect innovative changes in this field with the integration of Generative AI development techniques. This can help in terms of potential attack simulators, the creation of synthetic data for testing purposes of the security tools, and works on enhancing the solutions used for cyber threats.
2. Content Creation
This is the essential field for which generative AI development techniques are expanding rapidly. As in the content generation terms it works on generating content types like audio, video, and text formats. The content generation process mainly automates different tasks and lets the companies focus more on the complex and important tasks.
3. Healthcare
This is the economic sector that is required to be advanced frequently and integration of generative AI within these leads to changes like innovative drug designing, prediction of molecular behaviors, and generation of synesthetic medical records to efficiently train the AI systems. All these advancements are performed while taking care of patient policy for effective advancements in drug discovery and personalized medical experiences.
4. Manufacturing and Designing
This is the economic sector on which the whole business sector and the integration of generative AI development in this enhances many parts. The type of applications we can expect with GenAI in this field can be fully advanced supply chain management systems, automated manufacturing tasks, quality analysis units, multiple designing options, and waste material reduction.
Final Words
The changes we see with generative AI are not the same as we expect with the integration of other techniques. In other words, it holds transforming powers that are somewhere essential for bringing effective change. There might be some risks in its expansion but the advancement it provides can be beneficial in many terms. This article is all about exploring the nature of generative AI use cases and their expansion in the coming years. So give this a quick read to know more.