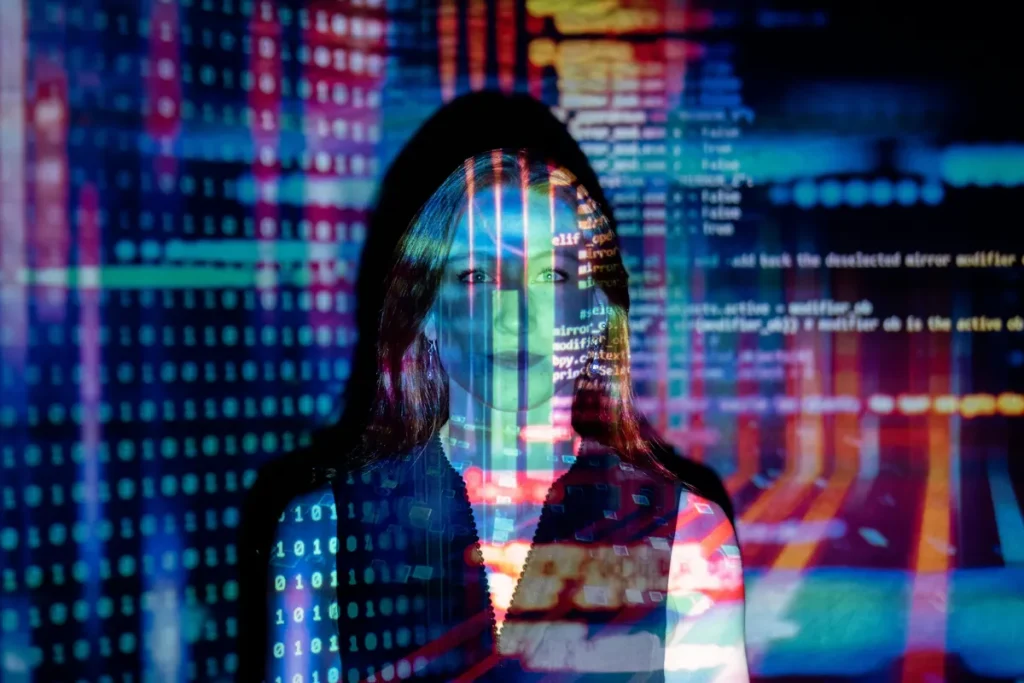
Data is today the lifeline of modern financial markets. As billions of data points are generated daily, making sense of this data is no mean task. Algorithmic trading has been an effective solution, employing data-led strategies to optimize trades, reduce risks, and maximize profits. Instead of relying on human intuition, algorithms read market patterns, identify trends, and execute trades with precision. Businesses and traders who harness the power of algorithmic strategies gain a competitive edge in today’s fast-paced trading environment. This article explores how algorithms turn raw data into actionable insights that drive profitability.
1. Data-Driven Decision Making: The Core of Algorithmic Trading
In financial markets, every second counts. Algorithmic trading systems process vast amounts of data at lightning speed, identifying trends and patterns that are impossible to detect manually. They use historical and real-time information to decide in a split second, thus ensuring trades occur at the best available prices. By removing emotional biases from decision-making, algorithmic methods enhance consistency and efficiency. Data-driven traders are able to respond to movements in the market faster than human traders, and this gives a big advantage in competitive trading environments.
2. Quantitative Models: The Force Behind Market Analysis
Algorithmic trading is based on quantitative models, which are written in the form of mathematical formulas that describe complex market phenomena. Quantitative models utilize past price trends, volume movements, and volatility levels to predict future trends. Statistical techniques, machine learning, and probability theory are applied to optimize trader strategies for different market conditions. Compared to traditional trading strategies, where decisions tend to be based on subjective analysis, quantitative models offer a systematic approach that is more precise. With the right models in place, traders are able to develop algorithms that adapt to changes in the market and identify profitable opportunities with high precision.
3. Backtesting: Validating Strategies Before Implementation
Before an algorithm can be implemented for live trading, it must be tested for robustness. Backtesting allows traders to try out their strategies on past data, and it provides them with an indication of the possible risks and profitability. By simulating various market situations, traders can fine-tune their models and eliminate deficiencies prior to the actual money being on the line. Proper backtesting ensures the algorithm will perform at its optimum during all market regimes. Without testing, even extremely sophisticated strategies might not perform under live conditions, making backtesting one of the most critical aspects of algorithmic trading success.
4. High-Frequency Trading: Speed as a Competitive Advantage
High-frequency trading (HFT) is the latest generation of algorithmic trading that executes thousands of trades per second. The very high-speed algorithms take advantage of extremely small price movements, profiting from market inefficiencies that are not visible to human traders. HFT firms employ advanced computing hardware and direct market access to trade with extremely low latency. As important as speed is an advantage, high-frequency trading must have robust risk controls in place to prevent costly errors. Firms that learn HFT gain a tremendous edge, capitalizing on opportunities that remain available for a fraction of a second.
5. Risk Management through Automated Execution
Algorithmic trading is not only about profit but also about minimizing the risk. Automated risk management functions are encoded in trading algorithms, and trades are carried out based on predetermined risk levels. Stop-loss orders, position sizing, and portfolio diversification are built into these systems to prevent excessive losses. Unlike human traders who might hesitate or make emotional decisions, algorithms mechanically apply risk management techniques. The disciplined trading approach ensures that losses are contained, saving capital even in volatile market environments. By combining automation with sound risk management principles, traders can safeguard their investments while maximizing returns.
6. The Future of AI in Algorithmic Trading
Artificial intelligence is revolutionizing algorithmic trading by making systems smarter and adaptive. Machine learning algorithms continuously refine their predictions with each new market data release, making them more accurate over time. AI-driven algorithms can capture subtle patterns, recognize anomalies, and modify trading strategies in real time. As financial markets become ever more complex, AI-based trading platforms will play an even more significant role in decision-making. The ability to sift through massive datasets and uncover hidden potential makes AI an indispensable tool for traders who want to stay ahead in an increasingly automated landscape.
Conclusion
Algorithmic trading has transformed financial markets, turning data into profitable opportunities through automation, quantitative models, and real-time analysis. Through the application of backtesting, high-frequency trading, and AI-driven strategies, traders can enhance efficiency and minimize risks. In a world where success is defined by speed and accuracy, those who embrace algorithmic trading have an unmistakable edge over the competition. The future belongs to traders who can combine technology with market expertise to ensure long-term profitability in an ever-evolving financial landscape.